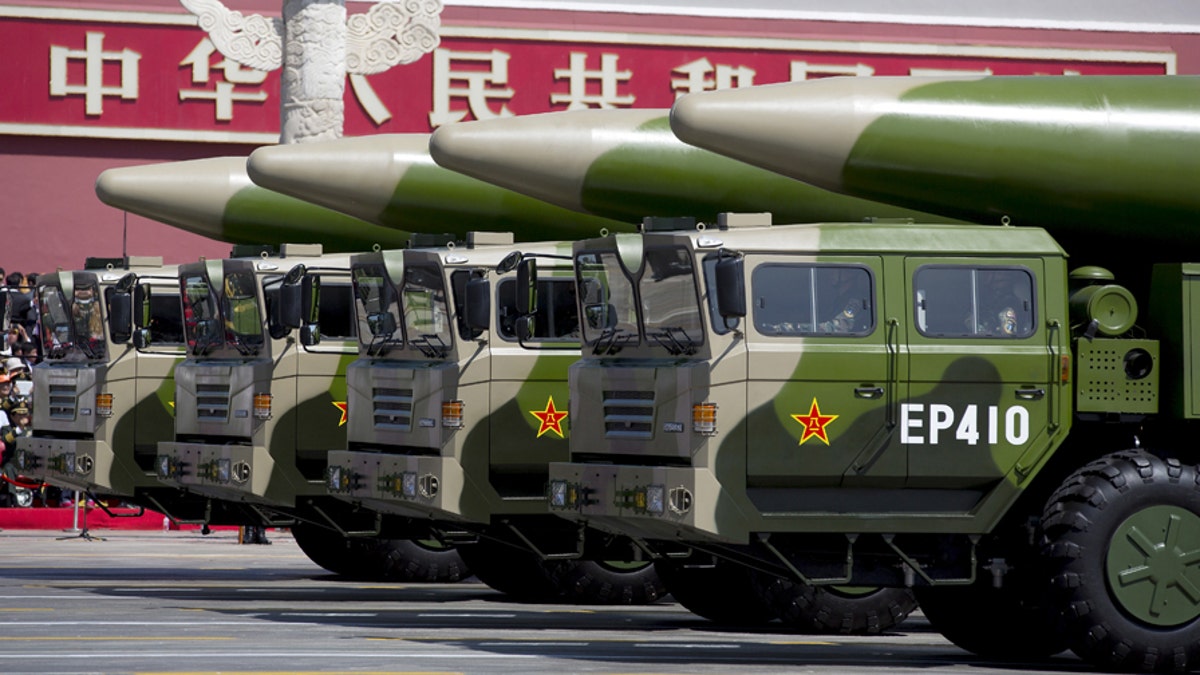
File photo - Military vehicles carrying DF-26 ballistic missiles travel past Tiananmen Gate during a military parade to commemorate the 70th anniversary of the end of World War II in Beijing Thursday Sept. 3, 2015. (REUTERS/Andy Wong/Pool)
U.S. researchers are using the same sort of deep learning artificial intelligence employed by Silicon Valley giants like Google and Facebook in their facial recognition technology to identify Chinese surface-to-air missile sites.
The deep learning algorithms used by researchers at the University of Missouri’s Center for Geospatial Intelligence were more than 80 times more efficient than a traditional human visual search in locating Chinese SAM sites, and were able to reduce the time it took to find these missile sites scattered across a nearly 90,000-square kilometer area of southeastern China from 60 hours to around 42 minutes.
Curt Davis, the director of Missouri’s Center for Geospatial Intelligence, told Fox News that while his work has so far only been employed at the research level, he believes it could be extremely beneficial to the military and government.
WILL THE US UNLEASH REVOLUTIONARY 'LIGHTINGSTRIKE' DRONE ON FUTURE ENEMIES?
“The Department of Defense already knows that they need to automate,” Davis said. “They now have more satellite imagery than their analysts can look over. This type of technology would free up a lot of their time to work on more difficult intelligence problems.”
Deep learning models, like GoogleNet and Microsoft’s ResNet, were originally created to detect and identify certain objects in photos and videos. Davis and his colleagues took these so-called “off-the-shelf deep learning models” and adapted them to deal with the challenges and limitations of satellite imagery.
For example, they trained some of these models to interpret both color and black-and-white images in case only black-and-white satellite images are available.
WHAT IS THE NUCLEAR ARSENAL AVAILABLE TO PRESIDENT TRUMP?
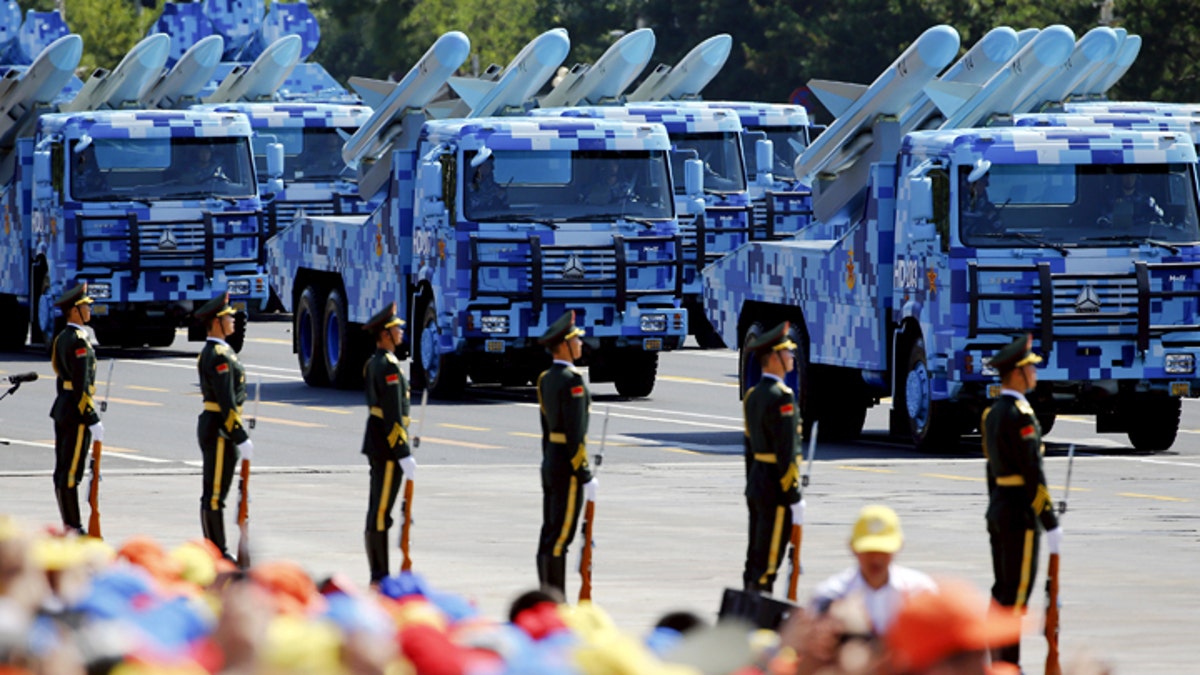
China's People's Liberation Army (PLA) navy soldiers on their armoured vehicles carrying ship-to-air missiles roll to Tiananmen Square during the military parade marking the 70th anniversary of the end of World War Two, in Beijing, China, September 3, 2015. REUTERS/Damir Sagolj - GF10000191226 (Reuters)
Davis admits that the algorithms sometimes wrongly identify missile sites, but still are able to match the 90 percent accuracy rate of human imagery analysts and, paired with humans going over the data, come up with fewer false positives.
“All machine learning creates false positives,” Davis said. “But the focus of the study was to measure the deep learning models against human performance.”
The area of southeastern China used by Davis and his colleagues is just slightly smaller than the entire country of North Korea, which has in the last year ramped up its intercontinental ballistic missile tests.
NORTH KOREA: WILL MINI NUKES BECOME AN OPTION FOR TRUMP?
In a special state media broadcast on Tuesday, North Korea said it successfully fired a "significantly more" powerful, nuclear-capable ICBM it called the Hwasong-15. Outside governments and analysts concurred the North had made a jump in missile capability.
A resumption of Pyongyang's torrid testing pace in pursuit of its goal of a viable arsenal of nuclear-tipped missiles that can hit the U.S. mainland had been widely expected, but the power of the missile and suddenness of the test still jolted the Korean Peninsula and Washington. The launch at 3:17 a.m. local time and midday in the U.S. capital indicated an effort to perfect the element of surprise and to obtain maximum attention in the United States.
While human analysts have possibly already located all of Pyongyang’s existing SAM sites, there is hope that the technology could help automatically locate any new sites.
POWERFUL TINY TANKS WITH VR AND FUTURISTIC COCKPITS COULD REVOLUTIONIZE THE BATTLEFIELD
“Knowing the location of existing and new SAM sites can sometimes lead analysts to other locations of interest, because countries often place SAM sites in specific areas to defend valuable nearby assets from air attack,” Wired Magazine reported.
Despite the glitches and false positives, Davis said that the study turned out better than expected and proved that deep learning methods can be applied on less-than-ideal satellite imagery.
“We wanted to test these deep learning methods on a realistic, real-world image analysis problem to critically assess their utility and potential impact,” Davis said. “The results were much better than we anticipated. Historically machine learning algorithms haven’t performed well when they have been applied to large satellite imagery datasets.”
The Associated Press contributed to this report.